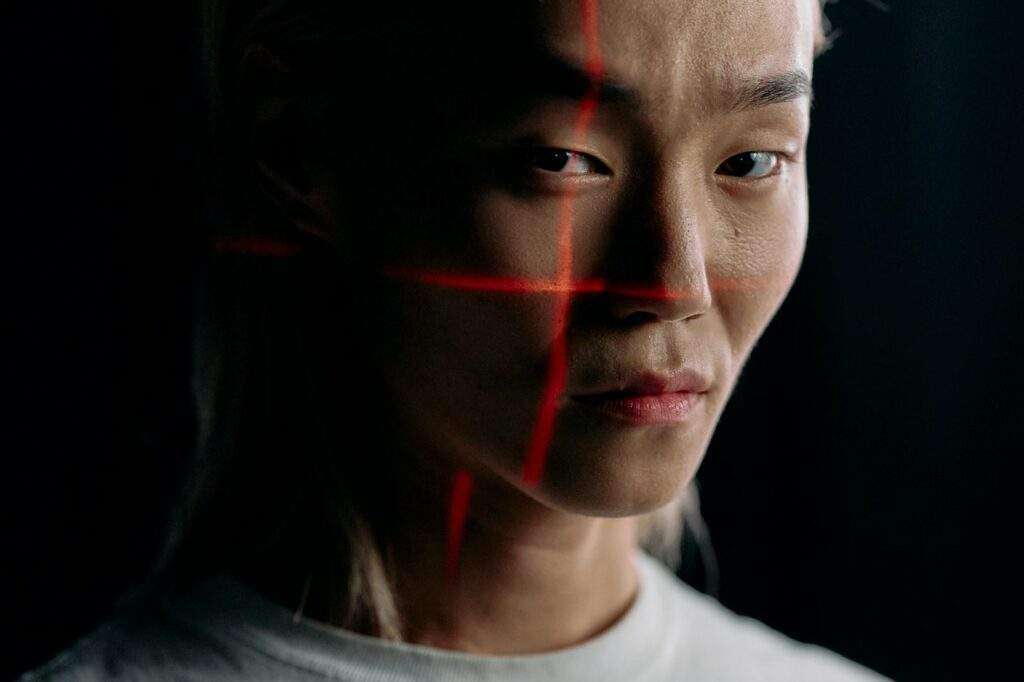
Facial recognition software has become increasingly prevalent in our daily lives, with applications ranging from unlocking smartphones to enhancing security systems. As this technology evolves, it is crucial to assess the accuracy and reliability of facial recognition algorithms. In this blog post, we will explore how one can determine the algorithm accuracy of facial recognition software, focusing on the use of FRVT assessment scores.
Understanding FRVT Assessment Scores:
FRVT (Facial Recognition Vendor Test) is a bench-marking program conducted by the National Institute of Standards and Technology (NIST) to evaluate and compare the performance of facial recognition algorithms. During the assessment, algorithms from various vendors are tested using standardized datasets and protocols. The results are then compiled into comprehensive reports, which include performance metrics and assessment scores.
Vendors can partake in algorithm testing and publicly share their FRVT assessment scores. It is to provide potential customers with an objective measure of the accuracy and effectiveness of their facial recognition algorithms. The scores indicate how well the algorithms perform across different scenarios, such as variations in lighting conditions, image quality, and pose. By examining these scores, users can gain valuable insights into the algorithm’s capabilities and limitations.
Interpreting FRVT Assessment Scores:
When analyzing FRVT assessment scores, it is essential to consider a few key metrics that provide a comprehensive overview of the algorithm’s performance:
- Identification Rate: This metric measures the algorithm’s ability to correctly match a given face against a database of known identities. A higher identification rate signifies better accuracy in identifying individuals.
- False Match Rate: The false match rate indicates the likelihood of a false positive. Hre the algorithm incorrectly matches a face to an incorrect identity. Lower false match rates are desirable, as they indicate a reduced risk of misidentifications.
- False Non-Match Rate: The false non-match rate measures the likelihood of a false negative. Here the algorithm fails to match a face to the correct identity. Lower false non-match rates imply higher reliability in recognizing individuals.
- Rank-1 Identification Accuracy: This metric represents the accuracy of the algorithm’s top match. It indicates the probability that the algorithm will accurately identify the correct person in the top-ranked position.
By examining these metrics and scores, users can gauge the algorithm’s performance under different conditions. They can compare it with other facial recognition software solutions.
Considerations beyond FRVT Assessment Scores:
While FRVT assessment scores provide valuable insights, they are not the sole factors to consider when evaluating facial recognition software. It is important to understand that these scores represent the algorithm’s performance on specific datasets and scenarios defined by the assessment process. Real-world usage might involve different conditions and variables that can impact the software’s accuracy.
Factors such as dataset bias, demographic performance disparities, and ethical considerations should also be taken into account. Evaluating the algorithm’s robustness against potential biases and addressing fairness and privacy concerns are crucial aspects of assessing facial recognition software. With all these various factors taken into account, you can click here to check out another vendor results example from an FRVT assessment
Conclusion:
FRVT assessment scores serve as an essential tool for understanding the algorithm accuracy of facial recognition software. They provide objective metrics that measure identification rates, false match rates, false non-match rates, and rank-1 identification accuracy. However, it is crucial to interpret these scores in conjunction with other considerations. Such as dataset biases, demographic performance disparities, and ethical implications. As facial recognition technology advances, ongoing evaluation and transparency are vital to ensure its responsible and effective use in our society.